GPT4ALL vs Llama: Unveiling the AI Showdown : Emily Rosemary Collins
by: Emily Rosemary Collins
blow post content copied from Be on the Right Side of Change
click here to view original post
The world of machine learning language models is rapidly evolving, with various powerful technologies competing for the top spot. Two of the most noteworthy contenders in this arena are GPT4All and LLaMA.
GPT4All, originating from Nomic, is a comprehensive ecosystem for open-source chatbots that offers a versatile framework for training language models. On the other hand, OpenLLaMA is an initiative by OpenLM Research to create a non-gated version of LLaMa for both research and commercial applications.
GPT4All and LLaMA: An Overview
In this section, we will explore two popular large language models, GPT4All and LLaMA, discussing their key features and differences.
GPT4All
GPT4All, initially released on March 26, 2023, is an open-source language model powered by the Nomic ecosystem. This AI assistant offers its users a wide range of capabilities and easy-to-use features to assist in various tasks such as text generation, translation, and more.
As an open-source project, GPT4All invites developers to contribute to its ongoing improvement, helping to keep the model accessible and versatile. With the support of its community, GPT4All continues to grow and evolve, offering increasingly better performance with each update.
It’s worth noting that GPT4All has been compared to other language models like Alpaca and LLaMA, but it maintains its own unique strengths within the world of AI-powered language assistance.
LLaMA
LLaMA, or the Local Large Memory Access, is another powerful language model that has made its mark in the AI community. It uses fewer parameters than some of the larger models, such as the 13B, 30B, and 65B versions, yet still offers impressive performance.
LLaMA-based GPT4All is known for its simplified architecture that allows users to create local ChatGPT clones easily. This design feature makes it possible to implement LLaMA in a variety of platforms and applications, giving developers more flexibility in choosing their AI language model solutions.
Aside from GPT4All, LLaMA also serves as the backbone for other language models like Alpaca, which was introduced by Stanford researchers and is specifically fine-tuned for instruction-following tasks.
In conclusion, both GPT4All and LLaMA offer unique advantages in the realm of AI-powered language assistance.
Key Features
Performance
LLaMA and GPT4All are both powerful language models that have been fine-tuned to provide high-quality results for various tasks. LLaMA is considered Meta AI’s most performant LLM for researchers and noncommercial use cases. It focuses on being more parameter-efficient than large commercial LLMs, making it a competitive choice.
Meanwhile, GPT4All, with its LLaMA 7B LoRA fine-tuned model, aims to provide users with an efficient and optimized performance.
Open Source and Licensing
Both LLaMA and GPT4All are open-source projects, which encourage community collaboration and user contributions. LLaMA is developed by Meta AI, and its open-source nature allows developers to learn, contribute, and adapt the project to suit their needs.
GPT4All, on the other hand, is based on the Meta LLaMA and licensed under GPL-3.0 which means that it grants users the freedom to modify, distribute, and share their versions of the project, although some restrictions apply.
Ecosystem and Access
The ecosystems surrounding LLaMA and GPT4All offer users various resources and tools that can benefit their projects. LLaMA has an extensive GitHub star timeline and is actively being developed by Meta AI. This commitment to improvement and innovation demonstrates the vibrancy of the LLaMA ecosystem.
In contrast, GPT4All’s ecosystem is centered around the usage of the LLaMA-based models for more specific applications. Users also have the option to create localized versions of ChatGPT, making it highly accessible for those who want to experiment with the technology.
Comparing Technologies
GPT Variants
GPT variants have evolved over time, starting from the initial GPT to the more recent GPT-3.5-Turbo and GPT-J. These models have progressively increased in size and capabilities. The GPT family offers powerful language generation and understanding with varying levels of performance, depending on the specific version.
LLMs
LLMs (large language models) have become the backbone of AI-based natural language understanding and generation. Models like LLaMA from Meta AI and GPT-4 are part of this category. LLaMA is a performant, parameter-efficient, and open alternative for researchers and non-commercial use cases. While GPT-4 offers a powerful ecosystem for open-source chatbots, enabling the development of custom fine-tuned solutions.
Nomics
The Nomic framework provides a platform for training LLMs with LLaMA and GPT-J backbones. Nomic AI fosters the creation of open-source chatbots and allows developers to leverage these advanced models for various applications. Leveraging the Nomic framework helps streamline the process of adopting large language models and their numerous benefits.
Practical Applications
When it comes to GPT4All and LLaMA, both language models have a range of practical applications, mainly in the areas of text generation, chatbots, and frameworks. In this section, we will discuss these specific applications in detail.
Text Generation
Both GPT4All and LLaMA excel in generating text for a variety of purposes. Whether you’re creating articles, translating languages, or writing creative content, these powerful models can produce coherent and informative output.
Due to their impressive performance in text generation, GPT4All and LLaMA have gained popularity among developers and businesses alike. Utilizing these models with Python-based setups allows for seamless integration into existing projects.
Chatbots
The open-source nature of GPT4All and LLaMA makes them ideal for chatbot development. These language models can carry out meaningful conversations with users, providing human-like responses and interactions.
Chatbots powered by GPT4All or LLaMA can be employed across industries, such as customer support, virtual assistance, and entertainment. By incorporating either of these models into chatbot applications, developers can significantly boost the quality and engagement of the AI-driven conversations.
Frameworks
Both GPT4All and LLaMA are supported by the Nomic framework, an ecosystem designed for open-source chatbots. This framework enables developers to train and fine-tune the models on a variety of backbones, including GPT-J.
By using platforms such as Meta AI, creators can harness the power of GPT4All and LLaMA to prototype, develop, and deploy AI chatbot solutions efficiently and effectively. The combination of these capable language models with state-of-the-art frameworks paves the way for advanced AI-driven applications.
Training and Fine-Tuning
When comparing GPT4All and LLaMA, it’s important to understand their training and fine-tuning processes, as well as the tools and services available for each.
OpenAI API and Finetuned Models
OpenAI, the creator of GPT-3, provides an API to access fine-tuned models like ChatGPT. These models benefit from reinforcement learning from human feedback (RLHF) , enabling them to be better aligned with the user’s expectations and use cases.
ChatGPT is a direct descendant of GPT-3, so users can access extensive documentation, resources, and community support when working with it.
Nomic and ALPACA Clients
In contrast, LLaMA is not directly available for commercial use, but developers can use Nomic – a framework for training LLMs with LLaMA and GPT-J backbones. Nomic allows access to various fine-tuned models like the Alpaca model, opening the doors for developers to experiment and improve upon their implemented solutions. Despite being smaller than commercial models, LLaMA has shown impressive performance in many benchmarks.
Research and Development
Academic Contributions
The development of both GPT4All and LLaMA has been driven by significant contributions from researchers and organizations in the field of natural language processing. Both models have their roots in technologies developed at Meta (formerly Facebook), with GPT4All being fine-tuned from the LLaMA 7B model.
Researchers from various institutions have contributed to the growth and fine-tuning of LLaMA and GPT4All. Their work has resulted in improved performance and scalability, allowing the models to be used in a variety of applications such as chatbots, content generation, and question-answering systems.
Benchmarks
To evaluate the performance of GPT4All and LLaMA, specific benchmarks have been established to measure their capabilities in terms of accuracy, language understanding, and responsiveness. One key factor in assessing performance is the hardware used to execute these models. For instance, Lambda Labs’ DGX A100 is a powerful computing platform that can provide valuable insights into the performance of large language models.
Comparing GPT4All and LLaMA on benchmarks such as the self-instruct evaluation offers a quantitative approach to determining each model’s effectiveness in processing and understanding languages. These benchmarks help users choose the best model for their needs, taking into consideration factors like execution time, cost, and deployment.
While GPT4All and LLaMA exhibit different performance characteristics, considering aspects such as academic contributions, benchmarks, and the advancements brought about by researchers and organizations like Facebook lead to a comprehensive understanding of these powerful language models.
Cost and Efficiency Considerations
When evaluating the performance of GPT-4All and LLaMA, cost and efficiency play an essential role in determining the most suitable LLM for a given use case.
M1 CPU Mac
Both GPT-4All and LLaMA aim to provide an efficient solution for users with varying hardware.
GPT-4All can be used on most hardware, including the M1 CPU Mac. Due to its simplified local ChatGPT implementation, it delivers quality performance on a variety of devices without sacrificing user experience.
LLaMA, on the other hand, is designed with higher performance in mind. Although it may perform well on an M1 CPU Mac, users might need to use hardware-specific compiler flags to optimize its performance for their specific devices.
Perplexity
Perplexity measures how well a model is able to predict test data — the lower the perplexity, the better the performance. Both GPT-4All and LLaMA have been fine-tuned to deliver high-quality LLM results.
GPT-4All offers cost-effective and fine-tuned LLM performance, which allows it to deliver high-quality results in a variety of applications.
LLaMA, on the other hand, boasts Meta AI’s most performant LLM available for researchers and noncommercial use cases. It’s designed to be a more parameter-efficient, open alternative to large commercial LLMs, even compared to GPT-3.5-Turbo.
In summary, both GPT-4All and LLaMA offer their own unique advantages in terms of cost efficiency, hardware compatibility, and performance metrics such as perplexity. Considering the specific needs of your project and available hardware will help you make the best choice between these two LLMs.
Future of Generative AI
AI Landscape
The AI Landscape is rapidly evolving, with new advancements taking place every day. Among the most promising technologies in this space are generative AI models like GPT4All and LLaMA. These models have the potential to revolutionize many industries, from content creation to design and beyond.
Free4All
The open-source nature of GPT4All and LLaMA-based systems provide an incredible opportunity for collaboration and innovation among developers and researchers. With increased accessibility to powerful generative AI models, the potential applications of AI technology will continue to grow and diversify.
One major benefit of such open-source approaches is the simplified local ChatGPT experience. This makes it easier for developers to create and utilize AI models on local machines without relying on cloud services or external dependencies.
MPT
Another important aspect of the future of generative AI is the development of MPT (Memory, Planning, and Transform) models. These models aim to combine the strengths of existing AI systems , such as GPT4All and LLaMA, while addressing their limitations. MPT models hold great promise for improving the overall performance of AI systems, enabling them to better understand context and accomplish complex tasks.
By incorporating memory and planning elements, MPT models can potentially offer even more powerful generative AI capabilities. As the AI landscape continues to evolve, the advancements in models like GPT4All, LLaMA, and MPT will significantly shape the future of generative AI and its impact on various industries.
Frequently Asked Questions
What makes GPT4ALL unique compared to LLaMA?
GPT4ALL is an ecosystem for open-source chatbots, built using LLaMA and GPT-J backbones. It aims to make large language models more accessible to developers, with an emphasis on running locally on your own CPU, resulting in reduced latency and privacy benefits.
How does LLaMA technology compare to GPT4ALL?
LLaMA (short for Large Language Model for Accelerators) is a project focused on making large language models more efficient on various computing devices using quantization methods. The LLaMA technology underpins GPT4ALL, so they are not directly competing solutions, but rather, GPT4ALL uses LLaMA as a foundation.
What are the advantages of GPT4ALL over LLaMA?
GPT4ALL provides pre-trained LLaMA models that can be used for a variety of AI applications, with the goal of making it easier to develop chatbots and other AI-driven tools. Also, GPT4ALL is designed to run locally on your CPU, which can provide better privacy, security, and potentially lower costs.
Can GPT4ALL perform tasks similar to LLaMA?
Since GPT4ALL is built on top of LLaMA technology, it can tackle many of the same tasks, such as natural language understanding and generation. However, GPT4ALL is more focused on providing developers with models for specific use cases, making it more accessible for those who want to build chatbots or other AI-driven tools.
How do GPT4ALL and LLaMA differ in performance?
GPT4ALL is designed to run on a CPU, while LLaMA optimization targets different hardware accelerators. This means that GPT4ALL models may have slightly lower performance than a native LLaMA implementation, but they offer advantages in terms of deployment flexibility and privacy.
What applications are best suited for GPT4ALL versus LLaMA?
Both GPT4ALL and LLaMA can be used for a range of Natural Language Processing tasks, such as text summarization or conversational AI. GPT4ALL is better suited for those who want to deploy locally, leveraging the benefits of running models on a CPU, while LLaMA is more focused on improving the efficiency of large language models for a variety of hardware accelerators.
June 27, 2023 at 04:01PM
Click here for more details...
=============================
The original post is available in Be on the Right Side of Change by Emily Rosemary Collins
this post has been published as it is through automation. Automation script brings all the top bloggers post under a single umbrella.
The purpose of this blog, Follow the top Salesforce bloggers and collect all blogs in a single place through automation.
============================
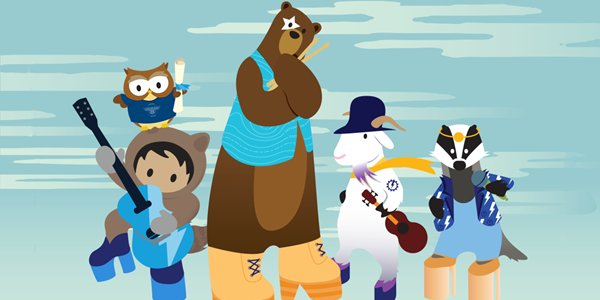
Post a Comment